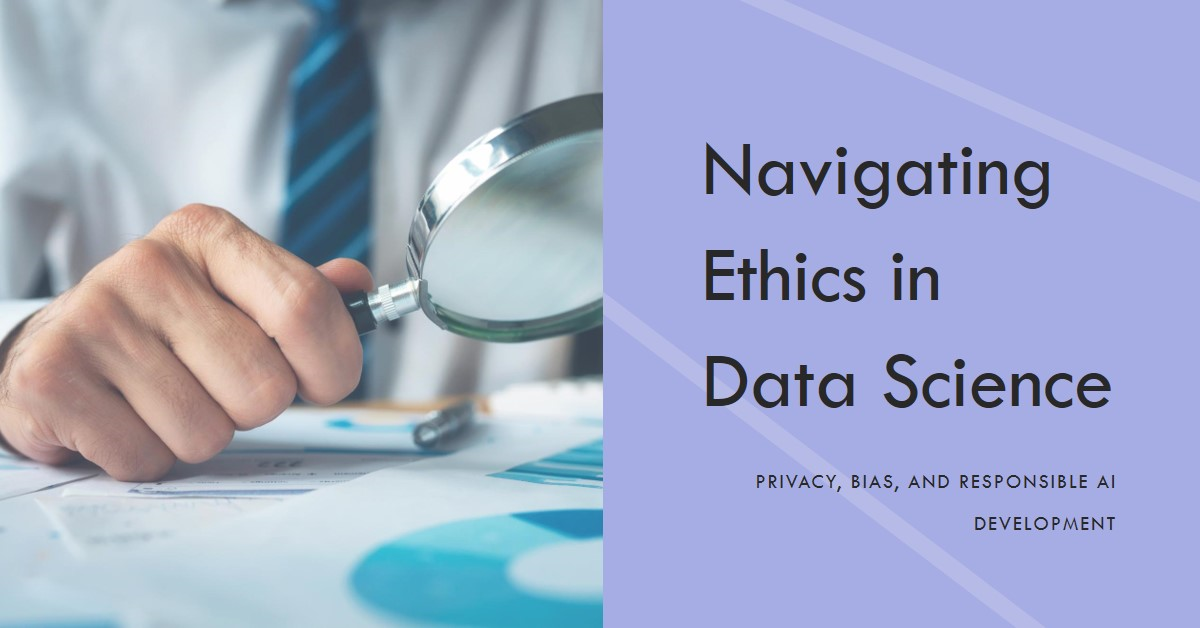
Ethics in Data Science: Navigating Privacy, Bias, and Responsible AI Development
In today’s digital age, the ethical implications of data science have become more significant than ever. From privacy concerns to biased algorithms and the development of responsible artificial intelligence (AI), navigating the ethical landscape is crucial for the future of the field.
I. Introduction
Definition of Data Science Ethics
Data science ethics refers to the moral principles and guidelines that govern the responsible conduct of data scientists, ensuring that their work aligns with societal values and norms.
Importance of Ethical Considerations in Data Science
The increasing reliance on data-driven technologies necessitates a focus on ethical considerations to protect individuals, communities, and society at large.
II. Privacy Concerns in Data Science
Overview of Privacy Issues
The widespread collection and analysis of personal data raise concerns about the invasion of individuals’ privacy and the potential misuse of sensitive information.
Impact of Data Collection on Privacy
Data collection practices, especially in the context of big data and machine learning, have a profound impact on privacy, necessitating a balance between innovation and safeguarding personal information.
Regulatory Frameworks and Compliance
Examining existing privacy regulations and compliance standards is vital for data scientists to ensure that their work adheres to legal and ethical standards.
III. Bias in Data Science
Understanding Bias in Algorithms
Bias in algorithms can result in discriminatory outcomes. Addressing this issue requires a comprehensive understanding of how biases emerge in data and models.
Real-world Consequences of Biased Models
Examining real-world consequences sheds light on the impact of biased models, emphasizing the urgency of mitigating bias in data science.
Strategies for Mitigating Bias in Data Science
Implementing strategies, such as diverse dataset curation and algorithmic transparency, can help mitigate bias and create fairer data science practices.
IV. Responsible AI Development
Definition and Principles of Responsible AI
Responsible AI development involves adhering to ethical principles, including transparency, fairness, accountability, and inclusivity, throughout the entire development process.
Ethical Decision-making in AI Development
Data scientists play a crucial role in making ethical decisions during the development of AI systems, considering potential societal impacts and long-term consequences.
Transparency and Accountability in AI Systems
Creating transparent and accountable AI systems is essential for fostering trust among users and ensuring that AI technologies align with ethical standards.
V. Case Studies
Examples of Ethical Dilemmas in Data Science
Exploring real-world examples of ethical dilemmas provides valuable insights into the challenges data scientists face and the decisions they must make.
Lessons Learned from Ethical Failures
Analyzing ethical failures helps the data science community learn from past mistakes and develop strategies to prevent similar incidents in the future.
Success Stories in Ethical Data Science Practices
Highlighting success stories showcases positive instances where ethical considerations have led to impactful and responsible data science practices.
VI. The Role of Stakeholders
Responsibilities of Data Scientists
Data scientists bear the responsibility of upholding ethical standards and ensuring the ethical use of data in their projects.
Organizational Ethics in Data Science
Organizations must establish ethical guidelines and frameworks to guide data scientists and promote ethical behavior within the workplace.
Public Awareness and Advocacy
Raising public awareness about data science ethics and advocating for ethical practices contribute to building a responsible data science community.
VII. Challenges and Future Trends
Emerging Ethical Challenges in Data Science
As technology evolves, new ethical challenges may arise, requiring continuous reflection and adaptation of ethical standards.
Potential Solutions and Innovations
Exploring potential solutions and innovative approaches helps the data science community stay ahead of emerging ethical challenges.
The Future of Ethical Data Science
Anticipating the future of ethical data science involves envisioning a landscape where responsible practices are integral to the development and deployment of data-driven technologies.
VIII. Conclusion
Recap of Key Ethical Considerations
Summarizing the key ethical considerations underscores the importance of integrating ethical practices into every facet of data science.
Call to Action for a Responsible Data Science Community
Encouraging a call to action urges data scientists, organizations, and policymakers to collaborate in creating a responsible and ethical data science community.
Frequently Asked Questions
What is data science ethics, and why is it important? Data science ethics refers to the moral principles guiding the responsible conduct of data scientists. It is crucial for ensuring that data-driven technologies align with societal values and norms.
How do biased algorithms impact real-world outcomes? Biased algorithms can lead to discriminatory outcomes, affecting individuals and communities. Understanding these consequences is vital for mitigating bias in data science.
What are the principles of responsible AI development? Responsible AI development involves principles such as transparency, fairness, accountability, and inclusivity to ensure ethical practices throughout the development process.
Why is public awareness essential in promoting data science ethics? Public awareness is crucial for fostering a responsible data science community, as informed individuals can advocate for ethical practices and hold organizations accountable.
How can organizations establish ethical guidelines for data science? Organizations can establish ethical guidelines by developing frameworks that guide data scientists, promoting ethical behavior, and creating a culture that values responsible data science practices.