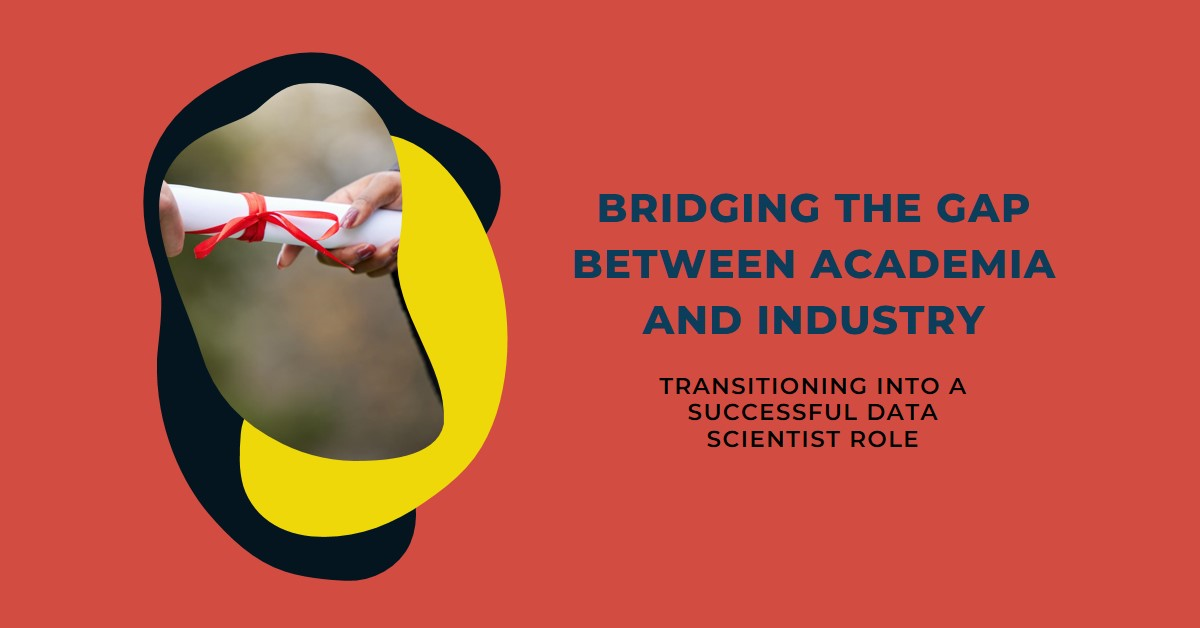
Bridging the Gap between Academia and Industry: How to Transition into a Successful Data Scientist Role
In today’s rapidly evolving technological landscape, the role of a data scientist has become increasingly pivotal. As businesses harness the power of data to make informed decisions, there’s a growing demand for skilled professionals who can bridge the gap between academia and industry. This article will guide you through the process of transitioning into a successful data scientist role, providing insights and practical tips to navigate the challenges.
I. Introduction
A. Definition of Data Scientist
The term “data scientist” often evokes curiosity. What does it mean to be a data scientist? In simple terms, a data scientist is a professional who gathers, analyzes, and interprets complex data to inform business decision-making processes.
B. Growing Importance of Data Science
Data science has transcended its niche and has become integral across various industries. As businesses embrace digital transformation, the need for skilled data scientists has surged, making it an exciting and lucrative career path.
II. Challenges in Transitioning
A. Academic vs. Industry Skill Gap
One of the primary challenges aspiring data scientists face is the gap between academic knowledge and real-world industry requirements. While academic programs provide a solid foundation, practical skills are often underestimated.
B. Real-world Application vs. Theoretical Knowledge
Theoretical knowledge is essential, but applying it to solve real-world problems requires a different set of skills. Navigating this transition can be daunting, but with the right approach, it becomes a rewarding journey.
III. Key Skills for Success
A. Technical Proficiency
Data scientists must excel in programming languages, statistical analysis, and machine learning. Continuous improvement in technical skills is crucial for staying competitive in the dynamic field of data science.
B. Business Acumen
Understanding the business context is as crucial as technical proficiency. A successful data scientist can translate data insights into actionable strategies that drive business success.
C. Communication Skills
The ability to convey complex findings in a clear and understandable manner is paramount. Data scientists often collaborate with non-technical stakeholders, making effective communication a valuable skill.
IV. Building a Strong Educational Foundation
A. Choosing the Right Degree
While a degree in data science or a related field is advantageous, it’s equally important to choose a program that aligns with your career goals and provides practical exposure.
B. Specialized Certifications
Supplementing your degree with specialized certifications can enhance your skill set and make you stand out in a competitive job market.
V. Gaining Practical Experience
A. Internships and Projects
Real-world experience is invaluable. Seek internships and work on projects that allow you to apply theoretical knowledge in practical settings.
B. Open Source Contributions
Contributing to open source projects not only builds your portfolio but also exposes you to collaborative environments, honing your teamwork and problem-solving skills.
VI. Networking and Industry Exposure
A. Attending Conferences and Meetups
Networking is a powerful tool. Attend conferences and meetups to connect with professionals in the field, learn about industry trends, and gain insights from experienced data scientists.
B. Utilizing Professional Networks
Platforms like LinkedIn offer opportunities to connect with professionals and join data science communities. Engaging in discussions and sharing your insights can broaden your network.
VII. Tailoring Your Resume
A. Highlighting Relevant Skills
Tailor your resume to showcase the skills and experiences most relevant to the data science role you’re pursuing. Use quantifiable achievements to demonstrate your impact.
B. Showcasing Real-world Projects
Instead of listing generic tasks, highlight specific projects you’ve worked on, detailing the challenges faced, methodologies applied, and outcomes achieved.
VIII. Mastering the Art of Interviewing
A. Technical Interviews
Prepare for technical interviews by revisiting key concepts, practicing coding challenges, and staying updated on industry-specific tools and techniques.
B. Behavioral Interviews
Behavioral interviews assess soft skills. Be ready to share examples of teamwork, problem-solving, and adaptability in previous roles.
IX. Navigating Job Platforms
A. Building an Impressive Profile
Craft a compelling online profile that reflects your expertise and passion for data science. Highlight your achievements, skills, and aspirations.
B. Actively Applying for Positions
Don’t wait for opportunities to come to you. Actively apply for positions, tailoring your applications to match the specific requirements of each job.
X. Staying Updated with Industry Trends
A. Continuous Learning
The field of data science is dynamic. Commit to continuous learning by staying updated on emerging technologies, tools, and methodologies.
B. Joining Data Science Communities
Engage with online communities, attend webinars, and participate in discussions to stay connected with the latest industry trends and advancements.
XI. Overcoming Imposter Syndrome
A. Embracing Continuous Growth
Imposter syndrome is common, especially in dynamic fields. Embrace continuous growth, understanding that learning is a lifelong journey.
B. Learning from Setbacks
View setbacks as opportunities to learn and improve. Each challenge is a stepping stone towards becoming a more resilient and capable data scientist.
XII. Success Stories and Inspirations
A. Profiles of Successful Data Scientists
Reading about the journeys of successful data scientists can provide inspiration and insights. Learn from their experiences and adapt strategies to suit your own path.
B. Learning from Others’ Journeys
Join online forums or attend webinars where successful data scientists share their experiences. Extract valuable lessons and apply them to your own journey.
XIII. Balancing Work and Continuous Learning
A. Time Management Tips
Balancing work responsibilities and continuous learning can be challenging. Implement effective time management strategies to maximize productivity.
B. Setting Realistic Goals
Set achievable and realistic goals for continuous learning. Break down larger objectives into smaller, manageable tasks to stay focused and motivated.
XIV. Conclusion
A. Summary of Key Points
Bridging the gap between academia and industry to become a successful data scientist requires a combination of technical skills, practical experience, and effective communication. Embrace the challenges, continuously learn, and stay persistent in your pursuit.
B. Encouragement and Motivation
The journey may be challenging, but remember that every successful data scientist started somewhere. Stay motivated, believe in your capabilities, and celebrate your achievements along the way.
XV. FAQs
A. How long does it take to transition into a data scientist role?
Transition times vary, but on average, it takes about 6-12 months with dedicated effort and continuous learning.
B. Are online certifications as valuable as traditional degrees?
Yes, many employers value relevant certifications, especially if they align with the skills required for the role.
C. How can networking help in the transition process?
Networking provides insights, mentorship, and job opportunities. It’s a valuable asset in navigating the transition.
D. What challenges do data scientists commonly face in their careers?
Challenges include staying updated with evolving technologies, managing large datasets, and effectively communicating findings to non-technical stakeholders.
E. How important is it to stay updated with the latest tools and technologies?
Staying updated is crucial in data science. New tools and technologies emerge, and staying current ensures you remain competitive in the field.