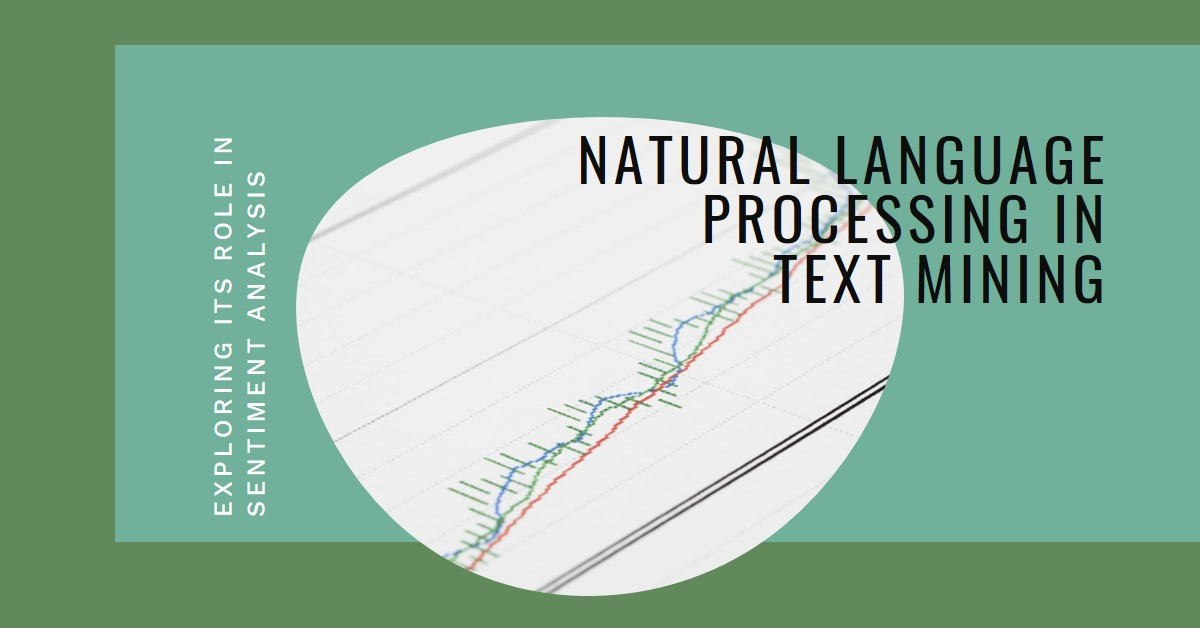
Exploring the Role of Natural Language Processing in Text Mining and Sentiment Analysis
Natural Language Processing (NLP) has emerged as a revolutionary technology, transforming the way we interact with machines and analyze vast amounts of textual data. In this article, we will delve into the intricate world of NLP, its crucial role in text mining and sentiment analysis, and the profound impact it has on various industries.
I. Introduction
A. Brief Overview of Natural Language Processing (NLP)
Natural Language Processing is a subfield of artificial intelligence that focuses on enabling machines to understand, interpret, and generate human language. It involves the development of algorithms and models capable of processing natural language data, making it accessible to computers.
B. Significance of NLP in Text Mining and Sentiment Analysis
In the era of big data, businesses are inundated with vast amounts of textual information. NLP plays a pivotal role in extracting valuable insights from this data through text mining and sentiment analysis. These processes enable organizations to understand customer opinions, track trends, and make data-driven decisions.
II. Understanding Natural Language Processing (NLP)
A. Definition and Core Concepts
At its core, NLP involves the application of computational models to the analysis and interpretation of human language. It encompasses a range of tasks, from basic language understanding to complex language generation.
B. Evolution of NLP Technology
Over the years, NLP has evolved significantly, driven by advancements in machine learning and deep learning. Modern NLP systems leverage neural networks and sophisticated algorithms to comprehend context, semantics, and sentiment in a way that mimics human understanding.
III. Text Mining: Unveiling the Basics
A. Definition and Scope
Text mining, also known as text analytics, involves the extraction of valuable information from unstructured textual data. This process includes tasks such as information retrieval, clustering, and topic modeling.
B. Key Components and Processes in Text Mining
Text mining comprises several key components, including text preprocessing, feature extraction, and machine learning algorithms. These components work in tandem to transform raw text into structured data, facilitating meaningful analysis.
IV. Sentiment Analysis: An Insight
A. Concept and Relevance
Sentiment analysis, or opinion mining, aims to determine the sentiment expressed in a piece of text. This can range from positive and negative sentiments to nuanced emotions, providing valuable insights into customer opinions and preferences.
B. Applications of Sentiment Analysis
Businesses across industries leverage sentiment analysis to understand customer feedback, monitor brand perception, and make informed marketing decisions. The insights gained from sentiment analysis contribute to enhancing customer experiences and building brand loyalty.
V. Synergies between NLP, Text Mining, and Sentiment Analysis
A. How NLP Enhances Text Mining
NLP enhances text mining by providing advanced techniques for language understanding. With NLP, text mining systems can better handle natural language nuances, improving the accuracy of information extraction and analysis.
B. Role of NLP in Improving Sentiment Analysis Accuracy
In sentiment analysis, NLP algorithms play a crucial role in deciphering context, sarcasm, and subtle nuances in language. This leads to more accurate sentiment classification, providing businesses with reliable insights into customer sentiments.
VI. Real-World Applications
A. Industry-Specific Use Cases
Various industries, including e-commerce, healthcare, and finance, have successfully integrated NLP, text mining, and sentiment analysis into their operations. Real-world applications range from customer feedback analysis to medical record processing and financial market sentiment tracking.
B. Success Stories and Case Studies
Examining success stories and case studies showcases the tangible benefits that organizations have realized through the strategic implementation of NLP technologies. These examples highlight the transformative power of NLP in diverse business contexts.
VII. NLP Challenges in Text Mining and Sentiment Analysis
A. Common Hurdles Faced
While NLP has made significant strides, challenges persist in areas such as context understanding, multilingual processing, and handling ambiguous language. Addressing these challenges is crucial for advancing the capabilities of NLP in text mining and sentiment analysis.
B. Ongoing Developments to Overcome Challenges
Researchers and developers continually work on addressing NLP challenges through innovations in algorithms, data preprocessing techniques, and model architectures. Keeping abreast of these developments is essential for organizations seeking to maximize the benefits of NLP technologies.
VIII. NLP Tools and Technologies
A. Overview of Popular NLP Tools
A multitude of NLP tools and libraries are available, catering to different needs and applications. This section provides an overview of popular NLP tools, highlighting their features and use cases.
B. Emerging Technologies Shaping the NLP Landscape
The NLP landscape is dynamic, with emerging technologies such as transformer models and pre-trained language models reshaping how NLP tasks are approached. Understanding these advancements is crucial for staying ahead in the rapidly evolving field of NLP.
IX. The Future of NLP in Text Mining and Sentiment Analysis
A. Predictions and Trends
Experts predict exciting developments in NLP, including improved language understanding, more accurate sentiment analysis, and increased efficiency in text mining. This section explores the future trends that will shape the landscape of NLP applications.
B. Potential Advancements on the Horizon
As technology continues to evolve, potential advancements in NLP include enhanced contextual understanding, better handling of complex languages, and increased personalization in text analysis. These advancements hold promise for further elevating the capabilities of NLP.
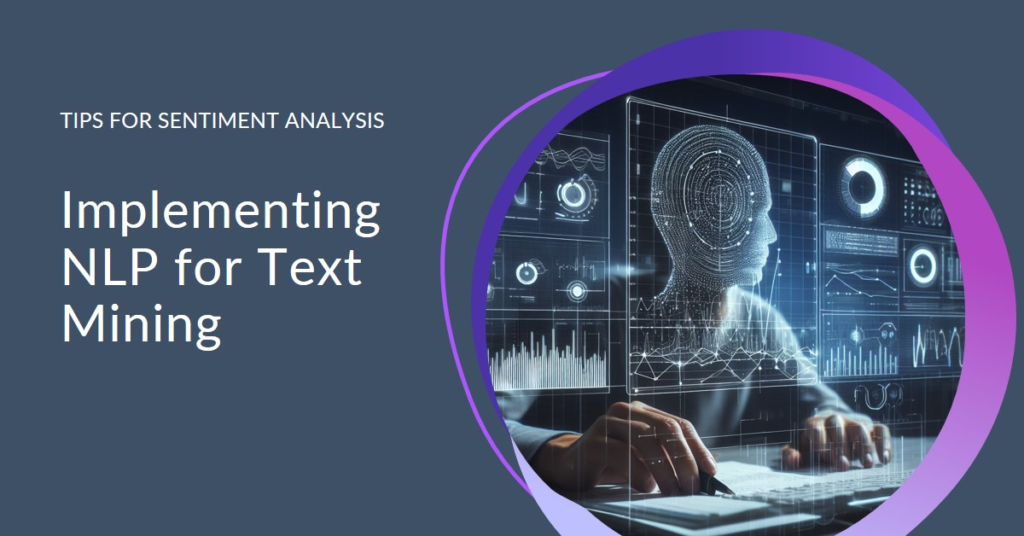
X. Best Practices for Implementing NLP in Text Mining and Sentiment Analysis
A. Tips for Seamless Integration
Successfully implementing NLP in text mining and sentiment analysis requires a strategic approach. This section provides practical tips for seamlessly integrating NLP technologies into existing systems and workflows.
B. Ensuring Accuracy and Reliability
Ensuring the accuracy and reliability of NLP applications is paramount. From quality data preprocessing to continuous monitoring and evaluation, adhering to best practices is essential for maximizing the benefits of NLP in text analysis.
XI. NLP and Ethical Considerations
A. Addressing Ethical Concerns in NLP Applications
As NLP technologies become more pervasive, ethical considerations come to the forefront. This section explores ethical concerns related to bias, privacy, and transparency in NLP applications.
B. Striking a Balance Between Innovation and Responsibility
Balancing innovation with ethical responsibility is a critical aspect of deploying NLP technologies. Organizations must navigate the ethical landscape to ensure that NLP applications benefit society while minimizing potential harms.
XII. NLP Adoption Challenges
A. Overcoming Resistance and Skepticism
Despite the proven benefits of NLP, organizations may face resistance and skepticism during the adoption process. Strategies for overcoming these challenges are crucial for successful NLP integration.
B. Building Awareness and Education
Educating stakeholders about the capabilities and benefits of NLP is essential for fostering understanding and support. This section provides insights into effective strategies for building awareness and educating teams about NLP.
XIII. Expert Insights: Interviews with NLP Professionals
A. Perspectives from Industry Experts
Interviews with NLP professionals provide valuable insights into the current state of the industry, ongoing challenges, and future trends. These expert perspectives offer a deeper understanding of the nuances and possibilities within the field of NLP.
B. Valuable Insights and Recommendations
Drawing on the expertise of NLP professionals, this section shares valuable insights and practical recommendations for individuals and organizations looking to leverage NLP in their operations.
XIV. Reader Engagement: Interactive Elements
A. Infographics and Visuals
Enhancing reader engagement is vital. Incorporating infographics and visuals helps convey complex concepts in a visually appealing manner, making the content more accessible and engaging.
B. Interactive Quizzes and Polls
Interactive elements such as quizzes and polls encourage reader participation. This section explores the use of interactive elements to foster engagement and gauge the understanding of key concepts.
XV. Conclusion
A. Recap of the Key Points
In conclusion, this article has explored the multifaceted role of Natural Language Processing in text mining and sentiment analysis. From understanding the basics of NLP to examining real-world applications and future trends, the journey through the intricacies of NLP highlights its transformative impact on how we process and analyze textual data.
B. Emphasizing the Role of NLP in Shaping the Future of Text Mining and Sentiment Analysis
As NLP continues to advance, its role in shaping the future of text mining and sentiment analysis cannot be overstated. Embracing NLP technologies and staying informed about industry developments will be key for organizations aiming to stay ahead in the evolving landscape of data analysis.
Frequently Asked Questions (FAQs)
What is Natural Language Processing (NLP)?
- Natural Language Processing is a subfield of artificial intelligence that focuses on enabling machines to understand, interpret, and generate human language.
How does NLP enhance sentiment analysis accuracy?
- NLP algorithms play a crucial role in deciphering context, sarcasm, and subtle nuances in language, leading to more accurate sentiment classification.
What are some common challenges in NLP applications?
- Common challenges in NLP include context understanding, multilingual processing, and handling ambiguous language.
What are the ethical considerations in NLP applications?
- Ethical considerations in NLP applications include issues related to bias, privacy, and transparency.
How can organizations overcome resistance to NLP adoption?
- Strategies for overcoming resistance to NLP adoption include educating stakeholders about the capabilities and benefits of NLP.